The false alerts eating your security budget and leaving you exposed to attack
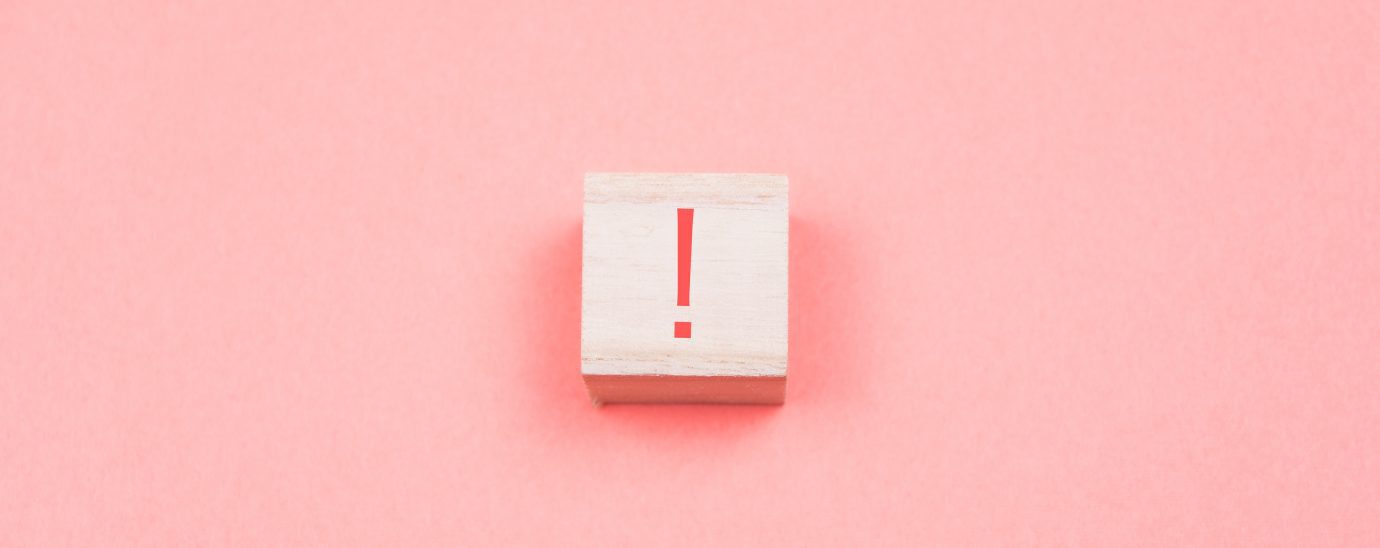
Security teams are increasingly inundated with thousands of alerts every day, most of which turn out to be false positives that represent no real threat to the organisation. Chuck Everette at Deep Instinct discusses how deep learning, the latest and most advanced form of AI analytics, can take on the avalanche of false positives – as well as tackling some of the most advanced cyber threats.
Imagine if just one in every thousand things on your to-do list was actually valuable to the business and your job function. Unfortunately, that’s the situation many security analysts find themselves in today as they are forced to deal with an ever-increasing amount of false-positive security alerts.
In fact, time-wasting false positives usually outnumber genuine alerts by far more than a thousand to one. In one instance, we encountered a large organisation that was generating around 75,000 alerts a day, of which on average only two related to real threats. While not always this severe, the issue is endemic across the business world. For example, deep Instinct’s Voice of SecOps report, which surveyed over 600 security decision-makers and practitioners around the world, found that security operation centre (SOC) teams are spending 10 out of every 39 hours in a working week dealing with false positives.
The huge number of alerts SOC teams must deal with everyday stems from the fact that security strategies usually revolve around equipping the organisation with an array of solutions that monitor every inch of the network for malicious activity. Anything suspicious or unusual that might be the sign of an attack in progress will be routed through a security information and event management (SIEM) platform and sent on to the SOC team to investigate, unfortunately often with little by way of context.
False positives appear when scanning tools lack sufficient fidelity to differentiate normal network traffic from suspicious activity. While the volume can be reduced by changing scanning sensitivity, this heightens the risk of genuine threats going unnoticed.
False positives impact security and personnel alike
The tidal wave of false positives presents a number of significant problems for organisations. Resolving a false positive is a necessary but very low-value activity that does nothing to improve the security of the business. The fact that SOC teams are spending a quarter of their time dealing with false alarms is extremely inefficient and stretches the time it takes for analysts to identify and investigate genuine threats.
Every second counts when a threat actor makes their move, so having a legitimate alert sitting in an inbox for days because it is lost amongst the noise is a serious issue, giving the attacker free rein to infiltrate the system and cover their tracks.
Spending so much time grinding through such a repetitive task also takes its toll on the security team and contributes to analyst burnout, a common problem in the industry. 90% of respondents in our survey stated they considered false positives to be contributing to low staff morale. Tired, demotivated SOC teams are also more likely to make mistakes, potentially missing the genuine threats hidden in the mountain of false positives.
Getting automated
With the volume of security alerts rising far beyond human capability, most companies have sought to automate at least some of the processes through artificial intelligence. This usually takes the form of machine learning (ML)-powered analytics tools that have been trained on data sets to recognise signs of different attacks.
At a basic level, these tools can quickly analyse large numbers of alerts and tick off all of the false positives, leaving the human team with a much more manageable workload. ML tools can also be integrated into response processes, enabling genuine but low-level threats to be resolved without human intervention. Similarly, threat investigations can also be heavily automated, blending human intuition with machine efficiency.
But while ML tools have proven to be invaluable in dealing with the alert avalanche, they are hampered by their reactive nature. Traditional solutions rely on incoming data feeds from endpoint detection and response (EDR) and other tools, which means they can only react to threats as they appear. Sophisticated threat actors have developed techniques that will strike and cause damage in the timeframe before ML tools have enough data to recognise the threat.
Further, some attackers are using their own machine learning tools to poison the well with falsified data sets that will confuse the solution into labelling signs of a real threat as ordinary network traffic. This allows attackers to infiltrate the network without being detected.
Countering this threat requires a more advanced form of AI analytics – deep learning (DL).
The next phase of AI
Deep learning is the latest and currently most advanced subset of AI. While it follows the same basic principle of ML, the key difference is that DL centres on a neurological network trained on raw, unlabelled datasets. Whereas ML tools are given datasets that are already differentiated into good and bad, a DL solution will learn to intuit the difference itself. The process is slower and more complex than traditional AI training but yields much greater results.
A fully trained DL network can instinctively identify subtle signs of malicious activity with a high degree of accuracy. Further, it does so at blistering speeds, even by the standards of AI – potential breaches can be identified in less than 20 milliseconds.
This combination of speed and accuracy means DL tools can identify incoming attacks at the very earliest opportunity, enabling security teams to shut them down before they can even begin swiftly. The more complicated training method is also much harder for threat actors to abuse with corrupted datasets.
DL is not some magical solution that will instantly resolve the false positive issue despite its advanced capabilities. CISOs will need to take their time assessing how best to assimilate the solution into their existing stack to optimise its capabilities.
Deep learning works most effectively when it is thoroughly integrated into the security stack, enabling it to deal with both genuine and false alerts as well as aiding in investigation and response to attacks. For example, we have found a well-integrated DL solution can deliver a 25% reduction in the number of alerts making it to the security team, and vastly speeds up the investigation of those that remain.
READ MORE:
- 5 questions businesses should ask in the wake of Biden’s cybersecurity bill
- Google employees to face vaccine mandate and remote work pay cuts
- Supporting your remote workforce: cloud migration for your business in five steps
- Using SAM to manage the security threat of remote working
With a fully trained and operational deep learning solution in place, security analysts will be able to get back to using their knowledge and skills on tackling the genuine threats facing their organisation, while their tireless machine ally takes on false positives and advanced attackers alike.
For more news from Top Business Tech, don’t forget to subscribe to our daily bulletin!