Deep learning: new neural nets could model continuous processes
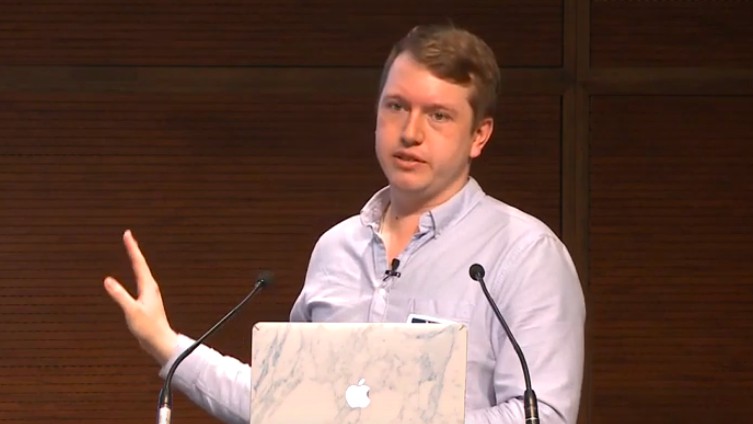
In deep learning, neural nets use specific hidden layers to deliver defined results. AI researcher David Duvenaud is questioning all of that with ODE nets.
Deep learning is incredible: truly, it is. Being able to map human-like brain power onto a computer, so that it learns as we do, should never be taken for granted. It is one of the most astonishing scientific breakthroughs in the history of our species, however, deep learning is not beyond improvement.
At the heart of a deep learning model lies a neural net. This is the brain, if you like: a combination of stacked layers of simple nodes that work to try and find the patterns in data. The net then assigns values to data that it processes, filtering this data through different layers to come to a final conclusion.
Now, scientists are questioning how the values are assigned to data and whether there’s a more efficient way to run deep learning algorithms.
David Duvenaud, an AI researcher at the University of Toronto, set out to build a medical deep learning model that would predict a patient’s health over a period of time. Traditional neural networks thrive when they learn from data with defined observation stages: basically, the hidden layers within a deep learning model. This is difficult to align with healthcare.
Health is a continuous topic to assess. It does not rely on binary questions as it contains so many variables. So how can a neural net pick up on continuous data?
Can neural nets be improved?
Think of a deep learning model as being similar to a game of classic board game, Guess Who. In the game, each player has a selection of characters in front of them, all with a different appearance: some have facial hair, glasses, blue eyes, brown eyes and each of them unique.
One player of Guess Who asks the other binary questions to discount characters from their investigation, until they are left with the final chosen character through this process of elimination: this is the output layer.
This is similar to how a neural network works. It processes its data through different stages, eliminating more and more of the dataset until it’s left with the correct answers available. This is the technology that is used in face recognition software, for example.
David Duvenaud saw an opportunity. He sought to break from the binary for a more fluid form of deep learning.
Traditionally, the answer is to simply add more layers to a neural net to reach a more accurate endpoint. This is not always sensible though. Why, for example, should you have to define the number of layers within a neural network, train the data and then wait to see how accurate it is? Duvenaud’s neural net lets you specify the accuracy first, then it finds the most efficient way to train itself within that margin of error.
This is what researchers describe as an “ODE net”, short for “ordinary differential equations”.
How can an ODE be solved?
Solving an ODE numerically can be done by integration. This is a computationally intensive task and there have been methods suggested in the past to reduce the hidden stages within deep learning.
Duvenaud worked with a number of researchers on a paper that proposed a simpler method to solve an ODE. The method relies on solving a second, augmented ODE backwards and doesn’t take up too much memory. The gradient computation algorithm works by introducing an “ODEsolve” operation as an operator later on in the process.
The ODE poses interesting questions about what the most efficient methods of deep learning truly are.
This operator relies on the initial state, the function, the initial time, the end time and the searched parameters from the ODE. The presented paper provided Python code to easily compute the derivatives of the ODE solver.
The paper suggested that supervised learning – particularly MNIST written digit classification – was one application in which the ODESolve method can perform compared to a residual network with much fewer parameters.
Will ODEs revolutionise deep learning?
The ODE is not the only way to run a deep learning model. There could be any number of reasons that a scientist would want to define the number of stages for the AI that they run. Either way, “it’s not ready for prime time yet,” Duvenaud claims.
However, the ODE poses interesting questions for deep learning moving forward about how we build neural nets and what the most efficient methods of deep learning truly are. This is not a particularly new idea, but this is a breakthrough of kinds. Whether this approach works for a range of models remains to be seen.