Our journey to hyper-personalization
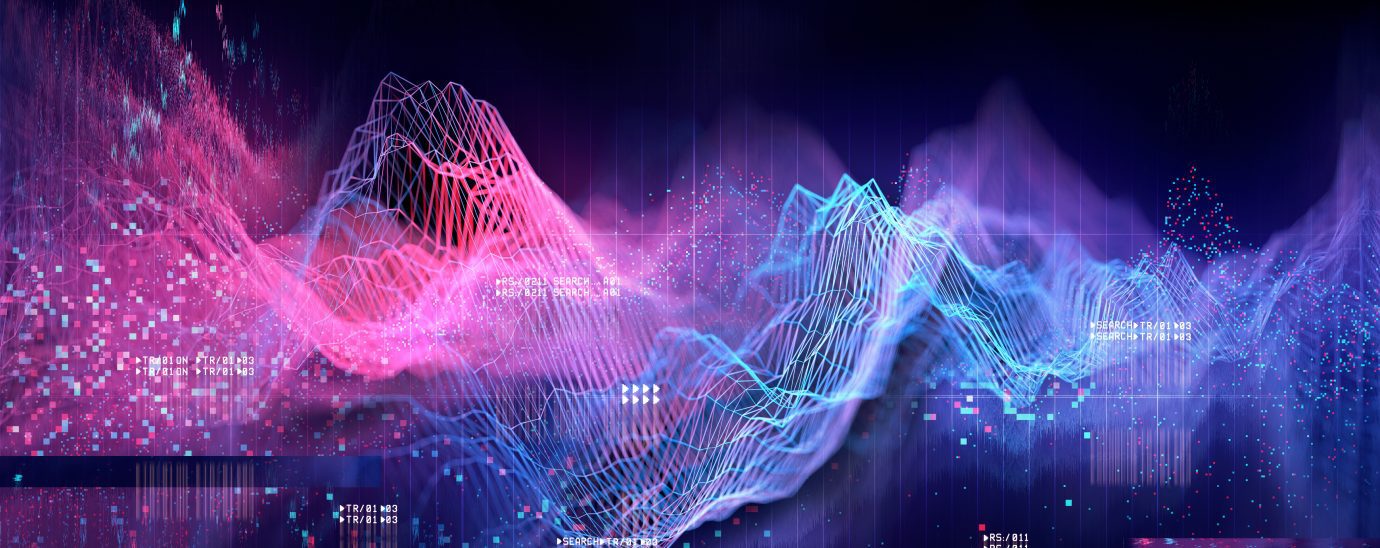
Paul Gwynn, CTO, FanFinders advises on how to achieve hyper-personalization.
Hyper-personalization is one of the key drivers behind the development of our new platform. Why? Because brands that create targeted, relevant and engaging experiences at every touchpoint with consumers are revolutionising marketing.
At the centre of this shift is data. Data that allows you to better understand who your customers are at every point of interaction. What are their unique interests? What preferences do they have? What defines them and the content they engage with? Once you have answers to these questions, it’s possible to build the intelligence to personalize experiences.
When done correctly, there is an opportunity to align these improved experiences for your users with more effective marketing. However, this is a complex and technical undertaking.
Training your AI
At FanFinders, we are fortunate to have access to a lot of historical data covering users, which in the parenting sector could be different ages, different stages in their pregnancy journey or different geo-locations. By using these various data points around our users, we can program an AI algorithm to achieve, for example, showing our members offers and content that is relevant for their current stage of pregnancy or where they live.
As we’re training the AI model, we’re aiming to get the correct weightings on the cause and effect of any individual data points; and perhaps just as crucial when engineering our data sets into the AI model is ensuring we don’t include any bias, either intentionally or by accident.
Finding the nuances
Using the data you have provided, the algorithm will pick out the best match. If we take offers in the parenting sector as the example, all of them could have up to five categories ranging from newborn, 3-6 months through to toddler. Alternatively, there could be topic categories like car seats, pushchairs, travel, feeding etc.
By applying specific attributes to the individual offer we have and seeing historically what people in the categories above selected, we can add these tags to any new offers on the platform and AI will rank relevance against the individuals demographics.
Why use AI? You could do this yourself via an algorithm, but the great thing about AI is that it can handle many variables, pick up the nuances within these data sets and react accordingly. The model should be able to take all data points into consideration and quickly analyse the permutations to select the best outcome.
Realistically, anything beyond a tiny set of data points and the human mind just can’t handle the permutation count in terms of either efficiency or accuracy.
Feeding the algorithm
It’s essential to get your data engineering correct. What I mean by this is if you have a raw date, for example: baby’s due date, this information is meaningless to the AI.
However, what you can do is engineer it so that when you’re providing this date, you’re classifying the baby’s age in months, which could be anything from -9 to 24 months. Now the AI can interpret the information efficiently and correctly. It’s shaping the data that you feed to the training algorithm in the right way.
During the training, outputs can also give you a sense of how well it has detected patterns in your data, which could be a range from 0-1.
0 would be it saying, “you gave me a million records to process but I’ll be damned if I can find any discernible patterns.” 1, by contrast, is the model saying it has found a concrete pattern.
What you’re seeking to do is get your model into the high 0.9s, where it can identify the data patterns your users are creating en masse, which would generally be invisible to humans.
The right level
While there is nothing unethical about showing your users more relevant content, these concerns should still be kept in the back of your mind as you build.
Using an AI algorithm to block or ban a user from a site is an example of a decision that you ultimately want being made by a human first.
There’s no reason you couldn’t use AI to highlight people for consideration, but we’ve specifically decided that actions that enforce restrictions on someone in their usage of an application should never be taken without human oversight.
Consumer expectations for personalization are rising and these must continue to inform the technology we build, finding the perfect level for it to thrive.
The type of personalization we are seeking for our platform is subtle but effective to the point where our users don’t necessarily realise when it’s happening – it doesn’t need to be in your face and we definitely don’t want it to make users uncomfortable.
READ MORE:
- Privacy versus personalization: a balancing act
- How technology can help consumers and businesses manage personal data online
- 5 Effective Ways of Personal Data Protection
- Experimentation plus personalisation: the magic equation for transforming business outcomes
We want to find intelligent ways to show users what is most of interest at the right times, provide recommendations and above all, build software that enhances their experience.
For more news from Top Business Tech, don’t forget to subscribe to our daily bulletin!