New methods of tackling Buy Now Pay Later (BNPL) fraudsters
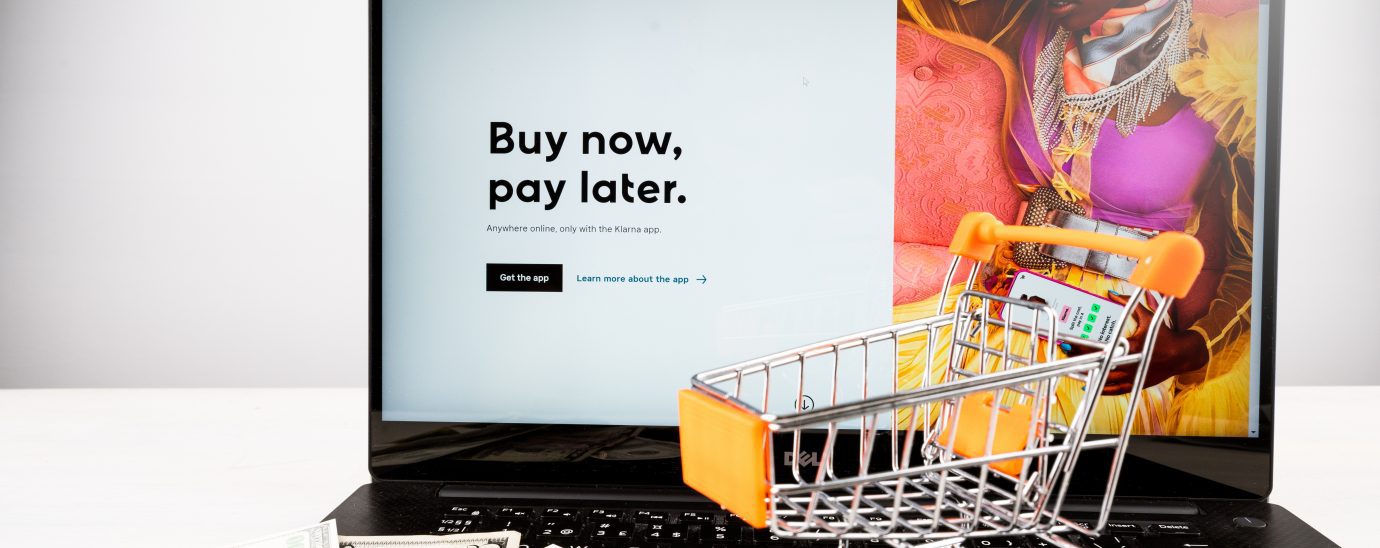
Martin Rehak, CEO of Resistant AI, discusses the new ways in which organizations can combat Buy Now Pay Later (BNPL) fraud.
Fraudsters are finding faster and more sophisticated ways of taking advantage of the increasingly popular Buy Now Pay Later (BNPL) services. While digital consumers enjoy the benefits of buying items they can’t necessarily afford immediately – from gift vouchers to mortgages – criminals are quick to find loopholes in this relatively straightforward process of instantly obtaining free credit online.
A surge in account takeovers
In 2020, we saw a reported 200% increase in account takeovers in digital commerce. In February 2021, the Compilation of Many Breaches (COMB) data breach exposed 3.2 billion users. Cybercriminals posted the victims’ accounts to a searchable online database that hackers and fraudsters could pay a small fee to access and create synthetic identities – where a criminal combines real and fake information to create a new identity – and commit first-party fraud for financial gain.
Account takeover can be particularly costly, especially when it involves established and reputable customers. Customer history and good reputation with a merchant is valuable, yet it can get stolen and misused. In the BNPL model, customers can secure approval in seconds and receive purchases having paid either nothing or a minimal amount upfront, a fraudster’s dream. These threats can result in additional measures to combat identity fraud, making the onboarding process cumbersome for the customer. What’s more, victims of identity theft can suffer from poor credit scores and identity validation issues, all of which needs to be avoided.
The BNPL provider’s conflict
A major conflict for BNPL providers lies in the balance of providing fast, simple service with a ‘soft’ credit check on consumers, versus protecting customer identities from criminals.
A further struggle is their reliance on top-drawer data by third parties as BNPL providers become dependent on multiple external data sources and services to validate their internal identification processes. And the more advanced the scoring, the greater their dependency on third parties. This exposes a gap for cybercriminals to fill.
Typical BNPL fraudster tactics
The BNPL fraudster will usually exploit:
- misconfigurations in the online merchant’s CRM
- vulnerabilities in the BNPL scoring code
- dormant, rarely used accounts to access long-forgotten passwords.
With each information leak of names, addresses, phone numbers and emails, there is one crime, in particular, that is really taking off. Attackers use synthetic identities to apply for finance and then order items that they ‘accidentally’ arrange to be delivered to an innocent victim’s home. The criminals then collect the package, leaving the unsuspecting victim with the bill.
The technical challenge for BNPLs
BNPL organizations need to challenge the increasing sophistication of cybercriminals with their own high-level tools to mitigate risk and counter known and unknown fraud attempts. Specifically, so they can improve their credit-scoring algorithms and protect fraud detection layers against manipulation and third-party gaps.
How can they do this?
A new breed of adaptive AI financial automation add-on
BNPL organizations can add a protective layer to their existing fraud detection systems. Today’s new financial automation oversight engines combine AI with advanced statistical and machine learning techniques to monitor underlying systems, expose fraudulent transaction patterns and improve the effectiveness of risk-based decision systems. They also continuously adapt to new fraud patterns so BNPL businesses can establish more robust controls across multiple platforms.
The new financial automation oversight engines can help in three critical areas:
1. Multiple algorithms combine to detect weak patterns to flag fraud
They find inconsistencies and high-dimensional correlations in data, and flag them for further investigation. This enables the BNPL organization to detect advanced fraud and manipulation earlier and faster. The ‘accidental’ delivery of packages mentioned earlier is less likely to happen. For example, the system will alert if an IP address cluster in Nottingham is being used to order products to multiple addresses in Bristol.
2.They identify previously unidentified vulnerabilities and third-party gaps
The new breed of automation engine will flag multiple types of misclassification. For example, it will create an alert if a gift voucher purchase, usually associated with low-risk items such as chocolates and soaps, is suddenly being used for high-risk electronic items. A high-level criminal could be planning to buy electronics elsewhere or sell the vouchers on eBay.
3.They can identify multiple transactions that don’t include a physical item
This suspicious activity will also flag a misclassification to the BNPL organization.
Happier customers, happier BNPL organizations
The result is good news for customers, who are less likely to become victims of fraud or bothered by unnecessary flags – which is an equally big plus for BNPL organizations since 40% of consumers say they won’t shop again with a merchant who falsely rejects their order.
READ MORE:
- Old Credit is History: Klarna’s new campaign
- How has 2021 become the golden age for fintechs?
- Mastercard to remove magnetic stripe from cards
- After the pandemic: the top 5 European e-commerce markets driving growth
It also improves the working lives of BNPL analysts, while BNPL organizations can sign up new customers quickly and confidently – customers who will have a trusted experience, boosting brand reputation and building a loyal customer base.
For more news from Top Business Tech, don’t forget to subscribe to our daily bulletin!